Quantitative Methods– Quantitative methods refer to approaches used in research and analysis that involve the measurement, quantification, and statistical interpretation of data. These methods are characterized by their emphasis on numerical data and the application of mathematical and statistical techniques to analyze and draw conclusions from that data.
Some common quantitative methods include:
- Descriptive Statistics: Summarizing and describing data using measures such as mean, median, mode, and standard deviation.
- Inferential Statistics: Making inferences and predictions about a population based on sample data, often using techniques like hypothesis testing and regression analysis.
- Experimental Research: Conducting controlled experiments to establish cause-and-effect relationships between variables.
- Survey Research: Collecting data through questionnaires or interviews to gather insights about attitudes, behaviors, or characteristics of a population.
- Modeling and Simulation: Creating mathematical models to simulate real-world scenarios and predict outcomes.
These methods are widely used across various disciplines, including social sciences, natural sciences, business, economics, and engineering, to provide rigorous analysis and evidence-based insights.
What is Required Quantitative Methods
“Required quantitative methods” typically refer to the specific quantitative techniques or approaches that are necessary or essential for conducting research or analysis within a particular field or study. The choice of required quantitative methods often depends on the nature of the research questions, the type of data available, and the goals of the study. Here are some examples across different disciplines:
- Economics: Econometrics techniques such as regression analysis, time series analysis, and input-output analysis are often required to analyze economic data and make forecasts.
- Social Sciences: Surveys, statistical tests (e.g., t-tests, ANOVA), and multivariate analysis (e.g., factor analysis, structural equation modeling) are common quantitative methods used to study human behavior, attitudes, and social phenomena.
- Health Sciences: Clinical trials, epidemiological studies, and biostatistics methods like survival analysis and logistic regression are essential for analyzing health-related data and making evidence-based decisions in medicine and public health.
- Engineering: Numerical methods, simulation techniques, and statistical process control are often required to design experiments, analyze data, and optimize processes in engineering and technology fields.
- Business and Management: Financial modeling, market research techniques (e.g., conjoint analysis, regression analysis), and operations research methods (e.g., linear programming, queuing theory) are crucial for making strategic decisions and improving organizational efficiency.
The choice of required quantitative methods may also be influenced by trends in research methodologies, technological advancements (e.g., use of big data analytics, machine learning), and the specific objectives of the study. Researchers often combine multiple quantitative methods to achieve comprehensive insights and robust conclusions.
Who is Required Quantitative Methods
“Quantitative methods” is not a person or a specific individual but rather a term used to describe a set of techniques and approaches in research and analysis. Quantitative methods refer to the systematic empirical investigation of observable phenomena via statistical, mathematical, or computational techniques. These methods are used to gather numerical data, analyze it statistically, and draw objective conclusions based on evidence.
In various fields such as social sciences, natural sciences, economics, engineering, and business, quantitative methods play a crucial role in:
- Data Collection: Using structured surveys, experiments, or observations to gather numerical data.
- Data Analysis: Applying statistical techniques (like regression analysis, hypothesis testing, and multivariate analysis) to interpret and draw conclusions from data.
- Modeling and Simulation: Developing mathematical models and simulations to understand complex systems and predict outcomes.
Quantitative methods are valued for their ability to provide precise measurements, test hypotheses rigorously, and generalize findings to larger populations or scenarios. They are essential tools for researchers and analysts seeking to uncover patterns, trends, and relationships in data to inform decision-making and advance knowledge in their respective fields.
When is Required Quantitative Methods
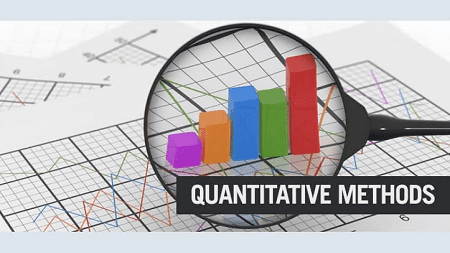
It seems like you’re asking about the timing or context in which quantitative methods are necessary or required. Quantitative methods are typically employed in research and analysis when there is a need to:
- Measure Phenomena: When researchers need to quantify and measure variables or attributes within a study.
- Test Hypotheses: When hypotheses need to be tested using statistical techniques to determine relationships or effects.
- Generalize Findings: When findings from a sample need to be generalized to a larger population.
- Predict Outcomes: When mathematical models or statistical analyses are used to predict future outcomes or behaviors.
- Ensure Objectivity: When there is a need for objective and systematic analysis of data.
The use of quantitative methods is often determined by the research questions, the nature of the data collected (typically numerical), and the goals of the study. Researchers decide to apply quantitative methods based on their research design and the specific insights they seek to gain from data analysis.
Where is Required Quantitative Methods
“Required quantitative methods” aren’t physically located anywhere specific because it’s a concept rather than a tangible entity or place. Quantitative methods refer to a set of techniques and approaches used in research and analysis, regardless of where the research is conducted. These methods can be applied in various settings such as:
- Academic Research: Universities, research institutions, and academic departments where researchers use quantitative methods to study various phenomena across disciplines.
- Business and Industry: Companies and organizations use quantitative methods for market research, financial analysis, operations management, and strategic decision-making.
- Government and Policy: Government agencies and policymakers use quantitative methods for economic forecasting, policy evaluation, and social research.
- Healthcare and Medicine: Quantitative methods are employed in clinical trials, epidemiological studies, and health services research to analyze health data and outcomes.
- Engineering and Technology: Engineers and technologists use quantitative methods for designing experiments, analyzing data, and optimizing systems and processes.
In essence, quantitative methods are used wherever there is a need to collect numerical data, apply statistical or mathematical techniques for analysis, and derive empirical insights or make informed decisions based on data-driven evidence.
How is Required Quantitative Methods
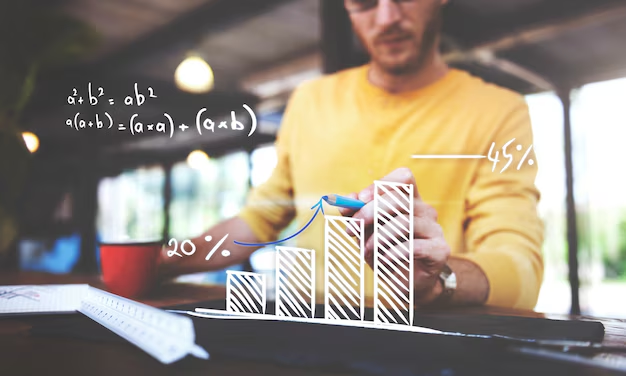
“Required quantitative methods” are utilized through structured approaches and techniques aimed at gathering numerical data, applying statistical analysis, and drawing objective conclusions. Here’s a breakdown of how these methods are typically applied:
- Data Collection: Quantitative methods involve systematic data collection using methods such as surveys, experiments, or structured observations. This ensures that data is gathered in a consistent and reliable manner.
- Quantification: Numerical data is quantified to represent variables or attributes being studied. This can include measurements, counts, ratings, or other forms of numerical representation.
- Statistical Analysis: Once data is collected, quantitative methods employ various statistical techniques to analyze the data. This includes descriptive statistics (such as mean, median, and standard deviation) to summarize data and inferential statistics (like regression analysis, t-tests, ANOVA) to test hypotheses and draw conclusions.
- Interpretation: Results from statistical analysis are interpreted to understand patterns, relationships, or trends within the data. This step involves making sense of the statistical findings in the context of the research questions or objectives.
- Validation and Generalization: Quantitative methods aim to validate findings through statistical rigor and generalize conclusions to broader populations or contexts, where applicable.
- Visualization: Often, quantitative data is visualized using charts, graphs, or tables to present findings effectively and facilitate understanding.
- Objectivity: Quantitative methods emphasize objectivity by minimizing bias and ensuring that conclusions are based on empirical evidence derived from numerical data.
Overall, the application of required quantitative methods involves a systematic approach to data collection, rigorous statistical analysis, and interpretation aimed at answering research questions, testing hypotheses, and contributing to knowledge within a specific field or discipline.
Case Study on Quantitative Methods

Certainly! A case study on quantitative methods typically involves an in-depth examination of how quantitative techniques are applied in a specific research project or study. Here’s an example scenario of a case study focusing on quantitative methods:
Case Study: Impact of Online Learning Platforms on Student Performance
Background: A university wants to assess the impact of online learning platforms on student performance in undergraduate courses. They aim to understand whether students who use online resources more frequently achieve better academic outcomes compared to those who do not.
Research Objectives:
- Determine the relationship between the frequency of online platform usage (in hours per week) and students’ final grades.
- Identify any demographic factors (e.g., age, gender, academic background) that may influence this relationship.
Methodology:
- Data Collection:
- Surveys are distributed to students at the end of the semester, collecting data on their frequency of online platform usage and final grades.
- Demographic information such as age, gender, and previous academic performance is also collected.
- Quantitative Analysis:
- Descriptive Statistics: Calculate measures such as mean, median, and standard deviation for the frequency of platform usage and final grades.
- Correlation Analysis: Use Pearson correlation coefficient to assess the strength and direction of the relationship between platform usage and final grades.
- Regression Analysis: Conduct multiple regression analysis to determine how much of the variance in final grades can be explained by platform usage, controlling for demographic variables.
- Findings:
- Students who used online platforms more frequently tended to have higher final grades, with a statistically significant positive correlation observed.
- Regression analysis showed that after controlling for demographic variables, the frequency of platform usage remained a significant predictor of final grades.
- Demographic factors such as age and previous academic performance also influenced student outcomes, though to a lesser extent than platform usage.
- Implications:
- The findings suggest that increased usage of online learning platforms is associated with improved academic performance.
- The university may consider promoting the use of these platforms and providing support for students to maximize their benefits.
- Future research could explore specific features of online platforms that contribute most to academic success and investigate potential interventions to enhance student learning outcomes.
Conclusion: This case study illustrates how quantitative methods, including surveys, statistical analysis (descriptive statistics, correlation, and regression), and interpretation of findings, can be applied to investigate relationships and draw evidence-based conclusions in educational research. It highlights the utility of quantitative approaches in understanding complex phenomena and informing decision-making within academic institutions.
Such case studies demonstrate the practical application of quantitative methods in research, providing insights that contribute to knowledge development and evidence-based practices in various fields.
White paper on Quantitative Methods
Writing a white paper on quantitative methods can provide a comprehensive overview of their application, benefits, and examples across different disciplines. Here’s an outline to guide the creation of a white paper on quantitative methods:
Title: Understanding Quantitative Methods: Applications and Insights
Introduction
- Overview of Quantitative Methods
- Definition and significance in research and analysis.
- Brief history and evolution of quantitative approaches.
Section 1: Foundations of Quantitative Methods
- Principles and Characteristics
- Systematic data collection and analysis.
- Emphasis on numerical data and statistical techniques.
Section 2: Key Techniques in Quantitative Analysis
- Data Collection
- Surveys, experiments, and observational studies.
- Descriptive Statistics
- Measures of central tendency and dispersion.
- Inferential Statistics
- Hypothesis testing, correlation, regression analysis.
Section 3: Applications Across Disciplines
- Social Sciences
- Case studies on demographic trends, survey research.
- Health Sciences
- Clinical trials, epidemiological studies.
- Business and Economics
- Market research, financial analysis.
- Engineering and Technology
- Simulation, modeling, optimization.
Section 4: Case Studies and Examples
- Case Study 1: Impact of Quantitative Methods in Market Research
- Use of regression analysis to predict consumer behavior.
- Case Study 2: Quantitative Approaches in Healthcare
- Application of clinical trials to evaluate treatment effectiveness.
Section 5: Benefits and Limitations
- Benefits of Quantitative Methods
- Objective and systematic analysis.
- Ability to generalize findings.
- Limitations and Considerations
- Assumptions underlying statistical techniques.
- Challenges in data collection and interpretation.
Section 6: Future Directions and Innovations
- Emerging Trends
- Big data analytics, machine learning applications.
- Ethical Considerations
- Privacy, data integrity, and transparency.
Conclusion
- Summary of Key Points
- Importance of quantitative methods in advancing knowledge.
- Recommendations for integrating quantitative approaches in research and decision-making.
References
- Citations and Resources
- Academic papers, books, and reputable sources on quantitative methods.
Writing Tips:
- Clarity and Structure: Ensure each section flows logically, providing clear explanations and examples.
- Visual Aids: Use charts, graphs, and tables to illustrate key concepts and findings.
- Engagement: Include real-world examples and case studies to demonstrate practical applications.
- Accessibility: Define technical terms and concepts for readers unfamiliar with quantitative methods.
By structuring your white paper in this way, you can create a comprehensive resource that educates readers on the fundamentals, applications, and future trends of quantitative methods across various fields.
Industrial Application of Quantitative Methods
Quantitative methods find extensive application in industry, providing rigorous tools for decision-making, process optimization, and predictive modeling. Here are several key industrial applications where quantitative methods play a crucial role:
1. Operations Research and Management
- Optimization Techniques: Quantitative methods like linear programming, integer programming, and queuing theory are used to optimize production schedules, inventory management, and resource allocation.
- Supply Chain Management: Statistical methods and modeling help analyze demand patterns, optimize logistics, and minimize costs in supply chain operations.
2. Quality Control and Process Improvement
- Statistical Process Control (SPC): Using control charts, hypothesis testing, and variance analysis to monitor and improve manufacturing processes, ensuring consistent product quality.
- Six Sigma: Applying statistical techniques to reduce defects and variation in production processes, enhancing efficiency and customer satisfaction.
3. Financial Analysis and Risk Management
- Portfolio Optimization: Using quantitative models to construct and manage investment portfolios, balancing risk and return.
- Credit Scoring: Statistical models assess creditworthiness based on historical data, guiding lending decisions.
- Risk Assessment: Quantitative methods, such as Monte Carlo simulation, evaluate and manage financial risks in investment and insurance sectors.
4. Market Research and Consumer Behavior
- Conjoint Analysis: Quantitative technique to understand consumer preferences and optimize product features or pricing strategies.
- Market Segmentation: Cluster analysis and factor analysis help identify customer segments based on demographics, behavior, and preferences.
5. Engineering and Manufacturing
- Reliability Engineering: Quantitative methods predict equipment reliability and maintenance needs, optimizing uptime and reducing downtime costs.
- Simulation Modeling: Using discrete event simulation or agent-based modeling to simulate complex systems, test scenarios, and optimize manufacturing processes.
6. Predictive Analytics and Data Mining
- Machine Learning: Applying algorithms for predictive modeling, anomaly detection, and pattern recognition in industrial applications such as predictive maintenance and fault detection.
- Big Data Analytics: Quantitative methods handle large datasets to extract actionable insights for process improvement, customer behavior analysis, and operational efficiency.
7. Environmental and Energy Management
- Resource Allocation: Optimization models assist in allocating resources like energy, water, and raw materials efficiently.
- Environmental Impact Assessment: Statistical analysis helps evaluate and mitigate environmental risks and impacts of industrial activities.
8. Healthcare and Pharmaceutical Industry
- Clinical Trials: Designing and analyzing clinical trials using quantitative methods to assess treatment efficacy and safety.
- Epidemiological Studies: Statistical methods analyze health data to understand disease patterns, risk factors, and public health trends.
Benefits of Quantitative Methods in Industry:
- Data-Driven Decision Making: Enables objective decision-making based on empirical evidence and statistical analysis.
- Efficiency and Cost Savings: Optimizes processes, reduces waste, and enhances resource allocation efficiency.
- Risk Management: Identifies and mitigates risks through quantitative risk assessment and modeling.
- Continuous Improvement: Facilitates ongoing process improvement through data-driven insights and performance metrics.
Quantitative methods continue to evolve with advancements in technology and data analytics, offering industries powerful tools to enhance competitiveness, efficiency, and sustainability in a global marketplace.